Speaker Series
Speaker Series is an ongoing PIBBSS project featuring researchers from both AI Alignment and adjacent fields studying intelligent behavior in some shape or form. The goal is to create a space where we can explore the connections between the work of these scholars and questions in AI Alignment.
By default, our speaker series consists of virtual talks followed by questions and discussions, happening over Zoom. Past recorded talks can be seen on our YouTube page or in their separate playlists (2022) (2023) (2024).
Going forward, our speaker series will be grouped into two-week sprints, happening several times per year. The next time we run them will be in February 2024. You can find the speakers and registration links for each below, and you can add our Google Calendar to always know when the next events will happen..
Upcoming Talks:
Micah Carroll
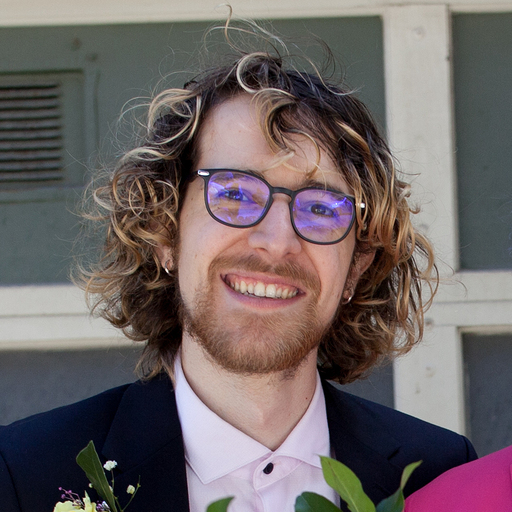
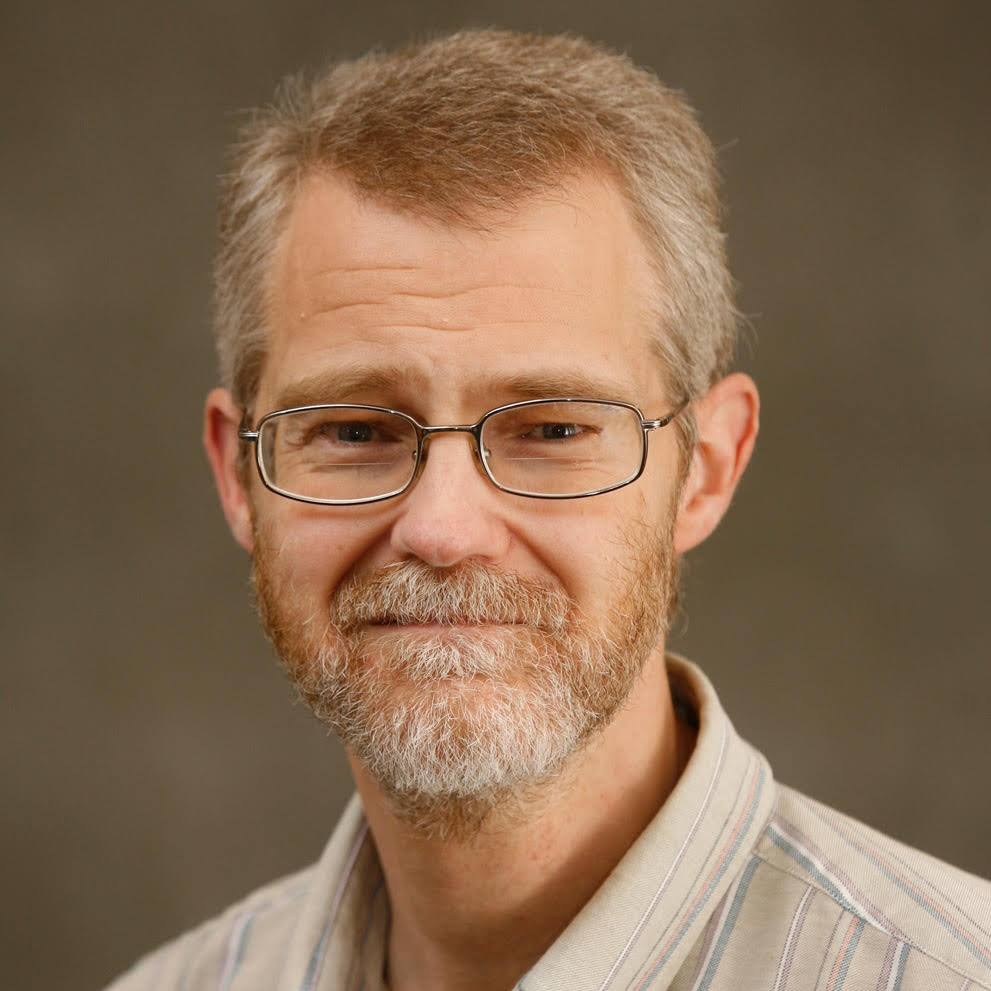
Randall D. Beer
July 15th, 16:00 UTC, 9:00 PDT, noon ET, 18:00 CET [Zoom Link]
Randall is a Provost Professor in the Cognitive Science Program, the Neuroscience Program, and the Dept. of Informatics at Indiana University. Broadly speaking, his research concerns how organisms operate as integrated wholes, with a particular focus on how behavior arises from the interaction between brains, bodies and environments. Toward this end, he works on the evolution and analysis of dynamical “nervous systems” for model agents, neuromechanical modeling of animals, biologically-inspired robotics, and dynamical systems and information theoretic approaches to behavior and cognition. He is also interested in computational and theoretical biology, including models of metabolism, gene regulation and development.
Autopoiesis and Enaction in the Game of Life
Enaction plays a central role in the broader fabric of so-called 4E (embodied, embedded, extended, enactive) cognition. Although the origin of the enactive approach is widely dated to the 1991 publication of the book “The Embodied Mind” by Varela, Thompson and Rosch, many of the central ideas trace to much earlier work. Over 40 years ago, the Chilean biologists Humberto Maturana and Francisco Varela put forward the notion of autopoiesis as a way to understand living systems and the phenomena that they generate, including cognition. Varela and others subsequently extended this framework to an enactive approach that places biological autonomy at the foundation of situated and embodied behavior and cognition. Unfortunately, these ideas have mostly been expressed purely verbally, making rigorously evaluate and debate. I will describe a research program aimed at placing these ideas on a firmer theoretical foundation by studying them within the context of a toy model universe, the Game of Life (GoL) cellular automata. This work has both pedagogical and theoretical goals. Simple concrete models provide an excellent vehicle for introducing some of the core concepts of autopoiesis and enaction and explaining how these concepts fit together into a broader whole. In addition, a careful analysis of such toy models can hone our intuitions about these concepts, probe their strengths and weaknesses, and move the entire enterprise in the direction of a more mathematically rigorous theory.
[Postponed] Seth Lazar
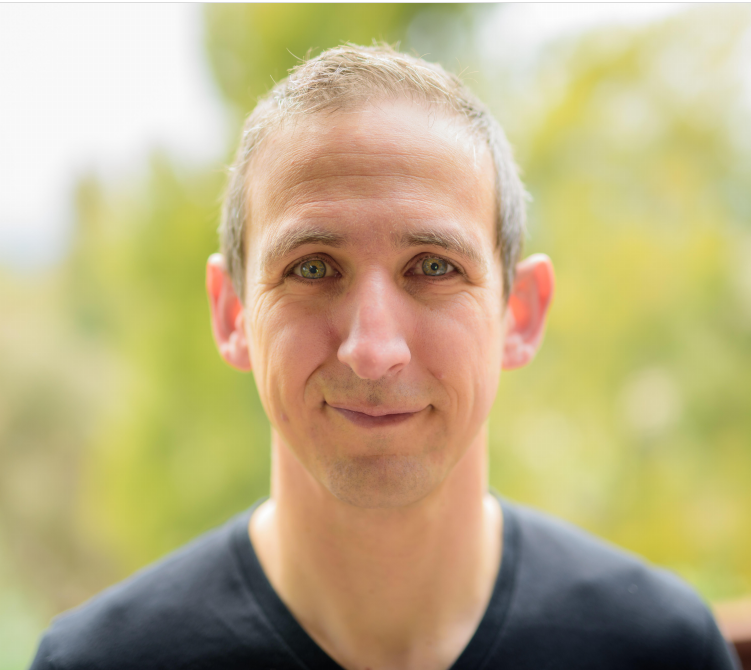
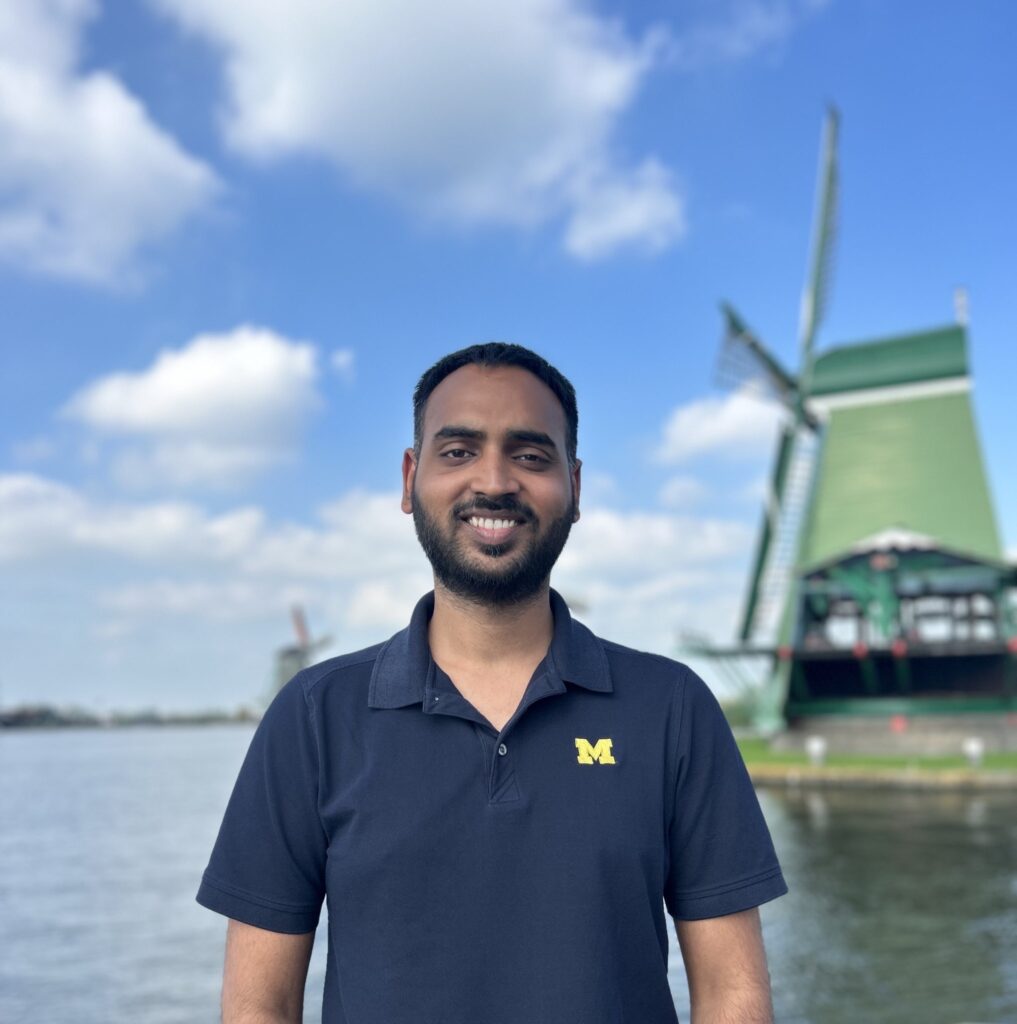
Ekdeep Singh Lubana
August 7th, 16:00 UTC, 9:00 PDT, noon ET, 18:00 CET [Zoom Link]
Ekdeep Singh Lubana is a postdoc at Center for Brain Science, Harvard University. Broadly, his research is focused on model systems for identifying novel challenges and better understanding existing challenges in alignment of AI systems. His recent work has revolved around developing mechanistic explanations for emergent capabilities in neural networks and demonstrating the brittleness of fine-tuning based approaches (e.g., RLHF) for alignment.
Explaining emergence in NN with model systems analysis
A fascinating phenomenon often seen in modern neural networks’ training is the sudden emergence of certain capabilities with scale. Specifically, such capabilities seem to be inexistent in the model until a critical amount of compute, data, or model size is reached, showing consistently and controllably thereafter. Since most policy frameworks for AI regulation are grounded in risk regulation, emergent capabilities are a big hurdle for such frameworks: regulating models for capabilities that are not yet present seems likely to be challenging (if not impossible). In this talk, we borrow the approach of model systems analysis from natural sciences to develop mechanistic hypotheses for what leads to the sudden emergence of capabilities in neural networks, identifying several unrelated mechanisms for this effect. These mechanisms have characteristic signatures that indicate preemptive estimation of the scale at which said capabilities will be learned may in fact be feasible.
Rio Popper
August 12th, 16:00 UTC, 9:00 PDT, noon ET, 18:00 CET [Zoom Link]
Rio Popper is a Research Fellow at the Global Priorities Institute, University of Oxford. She is also a Ph.D. candidate in economics at Stanford and a J.D. candidate at Yale Law School.
Popper on Popper
This talk addresses Karl Popper’s epistemology and its implications for AGI and ML. It first introduces Popper’s epistemology in its historical context and spells out the influence the theory has had since—both in science and in philosophy—and the implications it has for AI. However, while Popperian epistemology does have important implications for AI, some later philosophers have misapplied the theory. This talk also criticizes those misapplications.
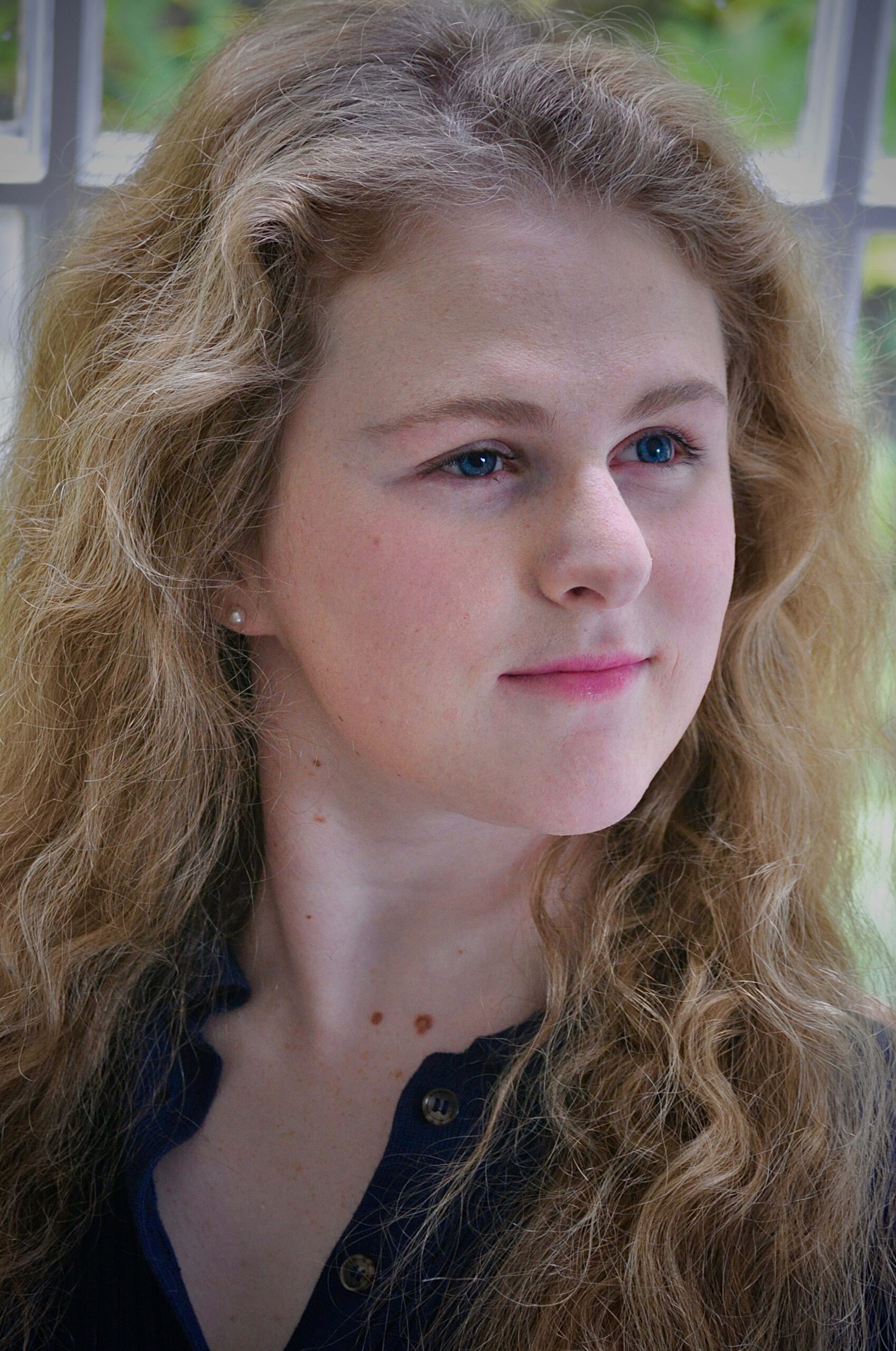
Past Talks:
Abram Demski
Recording can be found here
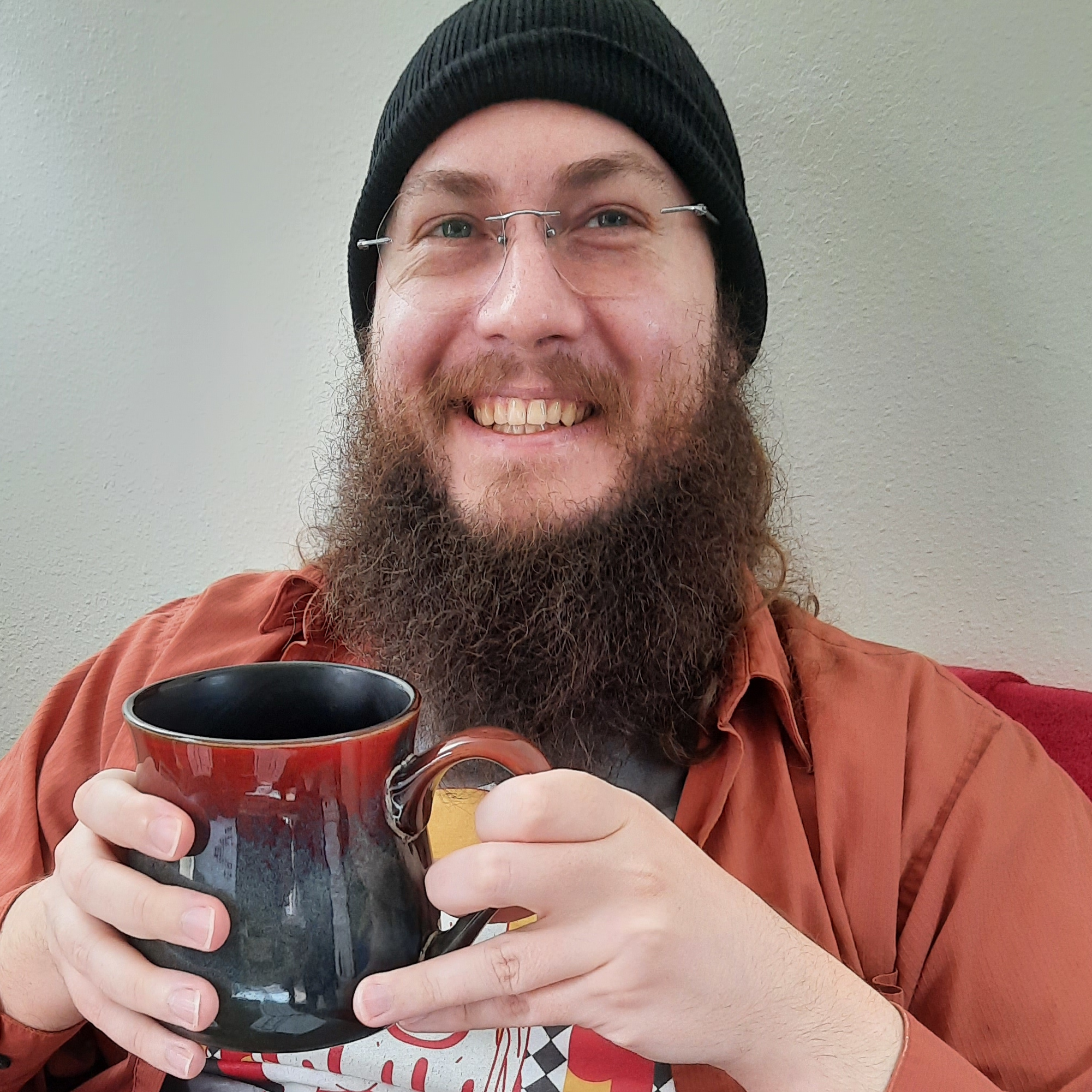
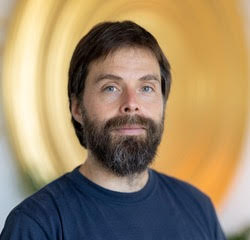
Fernando Ernesto Rosas De Andraca
Recording can be found here
Fernando is a lecturer at the University of Sussex, and a honorary research fellow at Imperial College London and University of Oxford. His research aims to develop a fundamental understanding the scope of interdependencies that can take place in systems involving many interacting parts, build practical algorithms to measure these in data, and apply these algorithms to fostering different forms of human well-being.
Towards a computational operationalisation of emergent phenomena
Emergence is one of the most fascinating and challenging aspects of complex systems in general and neural systems in particular, which let them feature unique properties at different spatio-temporal scales. While previous work has successfully developed tools to identify when emergent phenomena take place, they are limited in the degree they can specify how this happens. This talk introduces various formalisations of emergence, and put forward a theory that explains emergent phenomena in terms of their computational capabilities.
Daniel Polani
Recording can be found here
Daniel is a Professor of Artificial Intelligence at the University of Hertfordshire, UK. His interest is the modeling of cognition through the lens of information theory. His goal is to use the latter to identify a route to understand the principles of the emergence of intelligent cognition throughout evolution without the requirement of an external guide.
Information and its Flow: a Pathway towards Intelligence
In the last years, various forms of information flow were found to be useful quantities for the characterization of the decision-making of agents, whether natural or artificial. We discuss the consequences of the constraints on cognition imposed by information flow for the emergence of intelligent information processing, but also for the emergence of intrinsic incentives for behaviour, all expressed in informational language. It turns out that the informational perspective can yield surprising insights on how intelligent cognition might have been acquired throughout the course of evolution and, vice versa, how plausible intelligent abilities might be achievable with limited effort in artificial systems.
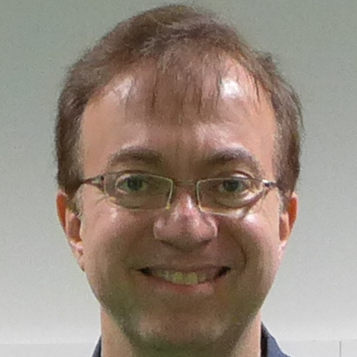
Tsvi Benson-Tilsen
Recording can be found here
Tsvi is employed by the Machine Intelligence Research Institute to figure out how to determine the underlying intentions of a hypothetical future AGI.
Creating the contexts needed to produce the concepts needed to understand minds
We are fundamentally confused about minds, and about what about a mind determines what about the world. Our concepts don’t automatically support the inferences and design choices we would like to make using those concepts, and there are strong forces that will break weak supports. Drive-by attempts to rework one or a few concepts in isolation don’t work. Minds are too big and structurally entangled within themselves to centrally unravel with a reductionist piecemeal method. The relevance of the most relevant mental elements is essentially provisional and requires the full context of a mind to be understood. The only source of usable data about minds and their intentions is our own minds. Within the context of our own minds and our familiarity with our own minds, we can maybe ask a network of questions that would induce ourselves to understand ourselves better enough–better enough that we could then understand how we could understand aliener minds well enough to design them to have agreeable intentions. We can’t survive while being as horrified as we are to try to understand how we work.
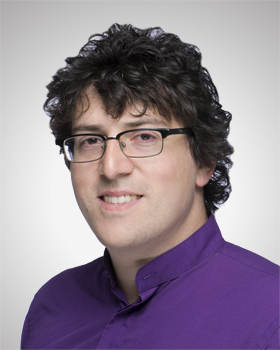
Nathaniel Virgo
Recording can be found here
Nathaniel Virgo is an interdisciplinary scientist with a background in mathematics, computer science, ecology and, more recently, applied category theory. He has a long-standing interest in the origin of life, and specifically the question of how something as complex and purposeful as life could emerge from a world in which it was initially absent.
Extending the classic “good regulator theorem” from control theory
The physical world appears, at first glance, to have things in it that are agents, in the relatively weak sense that they seem to have goals that they try to pursue, along with beliefs about the world that they update in the face of new information. Other things seem not to have these features. But where do beliefs and goals live in relation to the physical world, and why do some systems seem to have them while others don’t? I take the perspective that the difference between agents and non-agents is one of interpretation – goals are something that is attributed to a system by an observer, although some systems are more amenable to having goals attributed to them than others.
Along with my collaborators, I aim to make this idea mathematical, so that the process of attributing goals to a system can be made into a formal one, and the relationships between concepts like goals and beliefs can be fully understood. One ultimate goal is to understand why agent-like systems exist in the physical world at all. In particular, I will talk about extensions of the “good regulator theorem”, a classic result from early control theory that has been stated (somewhat inaccurately) as “every good regulator of a system must be a model of that system”. The original result concerned only fully observable systems, but using ideas from modern mathematics we extend it to a much broader class of systems that interact with their environments in much richer ways. The notion of ‘model’ also becomes richer, resembling Bayesian updating. One extension of the good regulator theorem could be stated as “every system that is a good regulator of itself must have a model of its environment”. The framework builds on recent ideas from categorical systems theory and is compositional in nature, allowing us to talk about multiple interacting systems. This leads to some interesting insights about the relationship between agents and their environments. We can conclude in particular that there is no unique place where the boundary between an agent and its environment should be drawn, although the interpretation in terms of beliefs might look quite different depending on the choice of boundary.
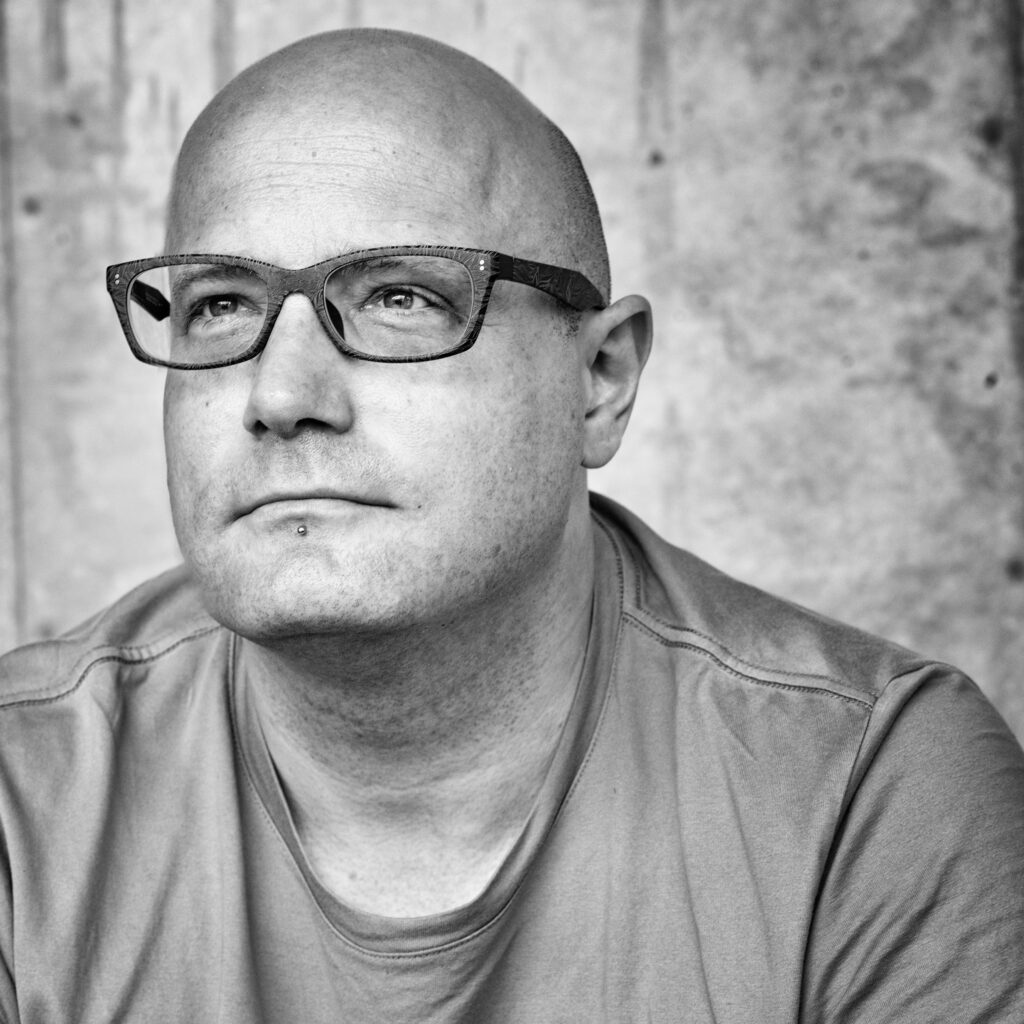
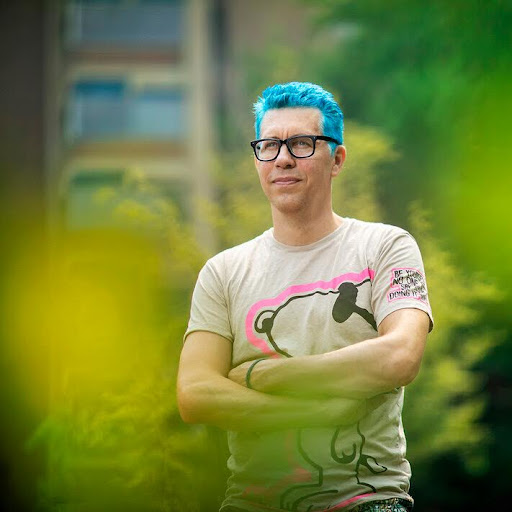
Konrad Paul Körding
Recording can be found here
Konrad is a German neuroscience professor at the University of Pennsylvania and co-founder of Neuromatch. He is known for his contributions to the fields of motor control, neural data methods, and computational neuroscience, as well as his advocacy and contribution to open science and scientific rigor.
On the interpretability of brains and neural networks
In my talk I will distinguish between approaches to infer or observe causality in nervous systems and approaches to understand these systems, approaches to make machine learning be understandable to human scientists. I will argue that progress requires a fundamental re-thinking of the goals of systems neuroscience.